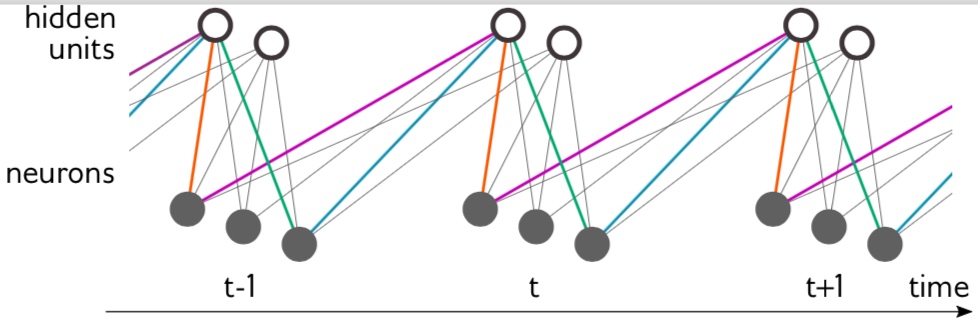
Biological systems pose new challenges for statistical physics compared to non-animate materials. The presence of strong, non-homogeneous interactions on many scales, coupled to non-equilibrium effects, makes standard first-principle-based modeling often ineffective. An attractive alternative approach is to directly infer effective descriptions, e.g. the interactions between the elementary constituents, directly from the data. Our team develops new methods inspired from statistical physics and machine learning to learn such models from biological data, and understand under which conditions these methods may be efficient. We apply our methods to various domains of biology, including gene regulation and development, neuroscience, immunology, genomics, and ecology, in close contact to experimental data and often in direct collaboration with experimentalists. The results of these data driven approaches in turn inspire novel phenomenological models.
The personal webpages of the members of the team can be accessed on the directory.